Determining the dynamical mass profiles of dispersion-supported galaxies is particularly challenging due to projection effects and the unknown shape of their velocity anisotropy profile. Our goal is to develop a machine learning algorithm capable of recovering dynamical mass profiles of dispersion-supported galaxies from line-of-sight stellar data. Traditionally, this task relies on time-consuming methods that require profile parameterization and assume dynamical equilibrium and spherical symmetry. We train a convolutional neural network model using various sets of cosmological hydrodynamical simulations of galaxies. By extracting projected stellar data from the simulated galaxies and feeding it into the model, we obtain the posterior distribution of the dynamical mass profile at ten different radii. Additionally, we evaluate the performance of existing literature mass estimators on our dataset. Our model achieves more accurate results than any literature mass estimator while also providing enclosed mass estimates at radii where no previous estimators exist. We confirm that the posterior distributions produced by the model are well-calibrated, ensuring they provide meaningful uncertainties. However, issues remain, as the method loses performance when trained on one set of simulations and applied to another, highlighting the importance of improving the generalization of ML methods trained on specific galaxy simulations.
Continuing this project, we explore the effectiveness of Graphical Neural Networks trained both in idealized and on cosmological hydrodynamical simulations. We find this type of neural network is able to extract dynamical information from stellar kinematics more efficiently than CNNs. We also highlight the suitability of GNNs for profiting from multiple tracer populations at once for dynamical inference.
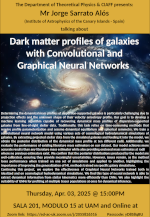